A recent UN survey showed that 85% of people around the world are concerned about misinformation. This concern is understandable. Dangerous conspiracy theories about ‘weather manipulation‘ are undermining proper management of hurricane disasters, fake news about immigrants eating pets in Ohio incited violence against the US Haitian community, false rumours about child kidnappings spurred deadly lynchings in India, and misinformation about health (such as ineffective cancer therapies) can have deadly consequences.
In a recent Skeptic article, Modirrousta-Galian, Higham, and Seabrooke recognise the dangers posed by misinformation but also argue that talk of ‘infodemics’ or comparing the spread of misinformation to that of a virus is a simplistic and misleading analogy that offers little but undue alarmism about the problem.
I believe this view is wrong, and predicated on a serious misunderstanding of the scientific literature. Most importantly, although the authors rely on several qualitative critiques of the analogy, they don’t actually engage with the rich mathematical, computational, and epidemiological evidence that illustrates why and how (mis)information spreads like a virus on social networks. This has absolutely nothing to do with alarmism, but rather with a proper understanding of the descriptive and predictive role of formal models in science.
For example, there are many dozens of empirical studies that use standard epidemiological models that are used to study how viruses propagate in a population to study how (mis)information diffuses in social networks. Importantly, these studies all find that such disease models fit social network data very well. This is an empirical fact.
One of the most well-known mathematical models of infectious diseases is the Susceptible-Infectious-Recovered (SIR) model where S stands for the number of susceptible individuals in the population, I for the number of infected individuals, and R for the number of recovered or resistant individuals. These models are typically generated from a series of straightforward differential equations and it’s not difficult to see how these models can be applied to the spread of misinformation where a susceptible individual encounters false information and subsequently propagates it to others in their network.
These models are incredibly useful because they allow us to predict and simulate population dynamics and derive epidemiological parameters such as the basic reproduction (R0) number (the average number of cases generated by an “infected” individual). And indeed, research finds that most social media platforms have an R0 greater than 1, indicating that the platforms have the potential for infodemic-like spread with some platforms having greater potential (eg Gab) than others. Similarly, other work shows how interventions, such as moderation or inoculation, could be usefully integrated in the ‘recovery’ compartment of the model to understand how interventions may reduce the spread of misinformation on social networks. Such models are validated with real-world social media dynamics.
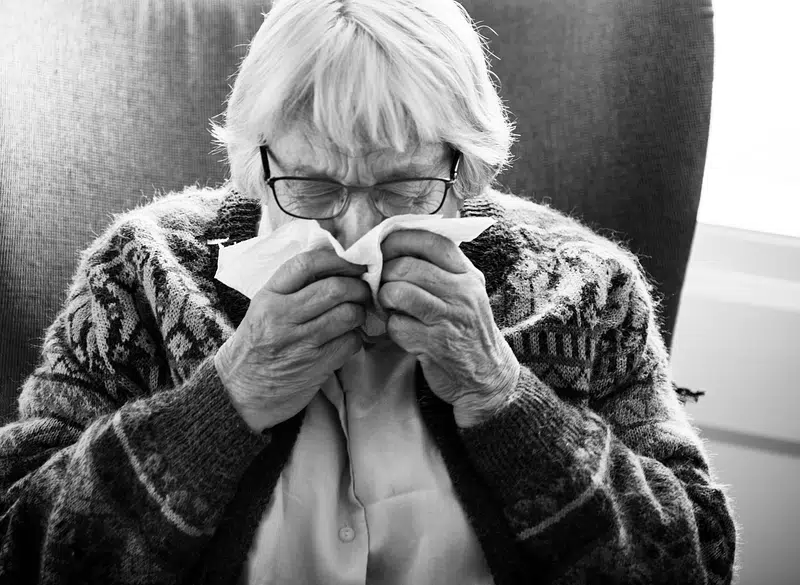
Modirrousta-Galian et al describe the analogy as “alarmist” because presumably the notion of infection following a single contact seems simplistic and implies anyone can become “infected”. However, that’s not a nuanced nor accurate view of either misinformation research or infection models. Studies reveal that people barely do better than chance when it comes to correctly identifying deepfakes. Moreover, it is trivial to show that repetition of false claims causes greater belief in those claims – an effect known as ‘illusory truth‘ – which impacts 85% of the typical sample, irrespective of the plausibility of the claim or prior knowledge that the claim is false.
The point is not whether people are gullible or not, but that some fake news stories clearly do spread like a simple contagion (eg fake rumours), infecting users immediately whereas others behave more like a complex contagion requiring repeated exposure from trusted sources (eg vaccine hesitancy). Critically, vaccine misinformation does not even have to convince to cause harm – it simply must induce fear, which we know gets more traction on social media.
Moreover, the fact that exposure effects can be cumulative, and susceptibility variable, does not detract from the usefulness of the abstraction, because these features are already present in many infection models, ranging from the simple to hugely complex. That all people are not equally susceptible to infection, or that some are virtually immune, or that sometimes you need to be sneezed on multiple times from a close distance to catch an infection, does not make the analogy less applicable. From a modelling perspective, this is a straight-forward matter of adjusting threshold parameters and relationships pertaining to population dynamics, reflecting how easy or hard it is for information pathogens to “infect” subpopulations.
Suggesting a pathogenic analogy for misinformation spread is alarmist misunderstands both the aptness of the analogy and the purpose of modelling. Mathematical modelling can be phenomenological (describing observed patterns) or mechanistic (making predictions based on known relationships) and both forms have demonstrated ample utility in misinformation research. Infodemiology is not just a term invented by the WHO – it’s an entire field of research with many accurate and useful predictions for how false information spreads and how interventions can be introduced to counter its spread. For example, prebunking or “psychological inoculation” interventions preemptively introduce and refute a weakened dose of a falsehood so that people gain immunity to misinformation in the future. Such interventions can be integrated in population models of misinformation spread.
Dismissing well-established analogies for modelling belief dynamics as an alarmist and misleading ‘metaphor’ without discussing the underlying science seems to have serious potential to misinform people on the topic. Misinformation does not occur in a vacuum – for a third of the US population to believe the 2020 election was “stolen”, or 37% to believe the FDA are suppressing a cure for cancer, requires beliefs so conceptually specific that they must evidently spread person-to-person, to our collective detriment. That not everyone is as readily susceptible does not negate this fact. Of course, although there’s a lot of mileage in the analogy, it’s not perfect. In the words of George Box, “all models are wrong but some are useful”.
If we really want to effectively tackle the spread of misinformation, we need all hands-on deck, including viral models that are both accurate and useful, not only for gauging where we stand, but to illuminate how we might counter the harms of misinformation in the future.