The expression “p-hacking” is relatively new to the science lexicon, but has caught on quickly. It is a label for various manipulations of data or analysis to achieve statistical significance when statistical significance was not truly earned. The term p-hacking ingeniously expresses the essence of these activities and provides a convenient shorthand for the growing discourse on the subject.
Producing novel, impactful research is how investigators get published, funded and promoted. It is also how medical entrepreneurs gather evidence and gain regulatory approval for their products. For better or worse, one of the factors that makes research more impactful is attaining findings that are “statistically significant.”
Statistical significance is considered a proxy for scientific validity or, in medicine, clinical significance. Studies with statistically significant results are more likely to published. When conducting research for regulatory approval (such as for the Food and Drug Administration), achieving statistically significant results can be essential for success.
The yardstick on which statistical significance is measured is known as a “p-value.” P-values has a very specific meaning:
The P value is defined as the probability under the assumption of no effect or no difference (null hypothesis), of obtaining a result equal to or more extreme than what was actually observed.
Although the definition is precise, a practical, intuitive understanding is elusive. Experts have difficulty explaining the concept of p-values to laypersons.
The pressure to create statistically significant research motivates some investigators to engage in p-hacking. They gently “massage” their data or tweak their analyses to transform a statistically insignificant result into a statistically significant one. These practices violate sound statistical principles, increase false positive results, and exaggerate real positive results.
P-hacking techniques may be employed naively by well-meaning investigators who believe they are polishing and presenting data in the best light. The motives may be innocent, but the consequence is the proliferation of false and exaggerated conclusions.
To understand p-hacking one needs a basic understanding of the null hypothesis, statistical testing, and p-values.
The null hypothesis
Medical research often looks for differences between variables or changes in variables over time. Do smokers have a greater risk of lung cancer than nonsmokers? Do diabetics treated with Drug A have lower blood sugars than those treated with Drug B? Do children raised in Springfield have higher IQs than children raised in Shelbyville?
Under most circumstances the statistical tests exploring these differences compare the measured results to a hypothetical assumption that there is no difference between the variables of interest. This assumption of no difference is known as “the null hypothesis.” The objective of research is then to collect data and run appropriate statistical analysis. If the results demonstrate a persuasive deviation from the null hypothesis the difference is declared to be “statistically significant.” If the measured difference between the test scores of Springfield and Shelbyville students is unlikely to occur under the null assumption, the difference is declared statistically significant.
Statistical testing and p-values
What differences are “persuasive enough” to reject the null hypothesis? This question is the essence of statistical testing. If we set the bar for persuasiveness too low, we risk rejecting the null hypothesis too easily, resulting in unjustified conclusions. We might incorrectly conclude that Springfield High students are smarter than Shelbyville students, when they are truly the same. This is known as a false positive (or Type 1) statistical error. If we set the bar for persuasiveness exceedingly high, we make it difficult to reject the null hypothesis even when real differences exist (a false negative, or Type II error).
P-values are expressed as decimals on a scale from 0 to 1. The p-value is the likelihood that a particular or a more extreme result would be obtained IF the null hypothesis is true. The smaller the p-value, the greater the inconsistency with the null hypothesis. Let’s pretend we run an experiment and measure slightly higher IQ for Springfield High students compared to Shebyville High. We run an appropriate statistical test and find a p-value of 0.45. This means that IF students in Springfield and Shelbyville have equal IQs, we would expect to find this particular result or a more extreme difference 45% of the time. For most purposes this would be insufficient evidence for rejecting the null hypothesis and we would not be confident declaring students in Springfield smarter than those in Shelbyville.
There are many reasons that a study might fail to reject the null hypothesis. It could be the null hypothesis is true, but it could also be a poorly designed study, or insufficient sample size, or a very small but real difference, or just bad luck. The p-value does NOT tell you how likely it is that the null hypothesis is really false or that the alternative hypothesis is really true. In most cases, it means there is insufficient information to make a reliable calculation of those propositions.
It is worth mentioning that failing to obtain a p-value low enough to reject the null hypothesis does not allow us to conclude that the null hypothesis is true. In other words, despite a high p-value, Springfield seniors might actually be smarter than their Shelbyville counterparts, but for whatever reason our experiment did not confirm this.
Before performing an experiment, researchers are obligated to define a threshold p-value. If the results of the experiment yields results that differ sufficiently from the null hypothesis to generate a p-value at or below the preselected threshold, the null hypothesis is “rejected” and the deviation from the null is declared statistically significant.
The p≤.05 standard
The selection of a threshold p-value is based on a variety of philosophical and practical considerations. Philosophically, we want to avoid false positive results – but there is a tradeoff. A very stringent threshold p-value decreases the chances of false positive findings, but effectively raises the hurdle to validate a truly positive finding. In other words, it also decreases true positive results and increases false negative results.
This can be overcome by designing larger, more powerful studies. Unfortunately, there are practical limitations to the funding and other resources for biomedical research, so larger studies are not always possible or practical. For better or for worse, a threshold p-value of .05 has become the de-facto standard for much of medical research. The consequence of a threshold p-value of .05 is that in situations where the null hypothesis is true, the research will erroneously reject the null hypothesis in 5% (1 in 20) of studies.
A dicey metaphor
Let us explore the implications of a threshold p-value of .05. P-values can range from 0 to 1. we can divide this range into 20 increments like this:
1. ≤.05 2.>.05-.10 3.>.10-.15, 4.>.15-.2 5.>.2-.25 6.>.25-.3 7. > .3-.35 8.>.35-.4 9.>.4-.45 10.>.45-.5 11.>.5-.55 12.>.55-.6 13. >.6-.65 14. >.65-.7 15.>.7-.75 16.>.75-.8 17.>.8-.85 18.>.85-.9 19. >.9-.95 20. >.95-1.0
Let’s then assign the upper limit of each of these increments to one side of a 20-sided die, like this one.
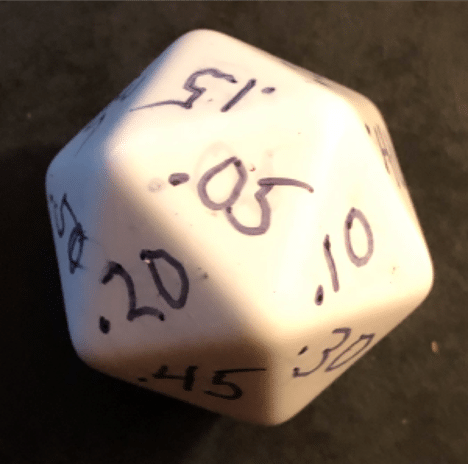
If we are comparing 2 groups that are, in fact, equal (the null hypothesis is true), utilising a threshold p-value of .05, every study is like a roll of that 20-sided die. One out of every 20 rolls of the die will land on the ≤.05 side. We will reject the null hypothesis and erroneously declare the two groups to be different.
Flexible sample size
When doing research, it is traditional to pre-specify the sample size (number of patients, specimens, test runs) for the study. Under ideal settings, this would be done based on existing clues about the behaviour of the groups being compared, and through the use of power calculations to ensure that the planned study has a reasonable chance of finding a real positive, if one exists. Often sample sizes are based on more practical considerations, such as the number of subjects available for study, funding, and other such limitations.
The p-hacker’s way to do it is to enrol a few patients, analyse the results, enrol a few more and repeat the analysis. This cycle is repeated until a statistically significant result is achieved. Then enrolment is halted. At first glance this seems like a very efficient way to do a study. Only the minimum number of patients needed to achieve statistical significance are needed.
Here’s the problem: if you want to minimise false positives, you have to roll die and accept the final lie. During the course of the roll, the die will inevitably roll over multiple sides before it ultimately comes to rest. By repeated enrolling and re-analysing, it is as if we take intermittent snapshots of the die in motion. If they happen to catch the die with the <.05 side face up, the die is stopped mid-roll, and victory is declared. In order to avoid excess false positives you have to set the parameters in advance and accept the outcome of the roll.
Slicing and dicing (also known as multiple comparisons and data dredging)
I can think of no better example of so-called data dredging than this gem from Randall Monroe’s xkcd cartoon. In his Great Jelly Bean study, authors report the shocking result that green jelly beans are linked to acne, complete with a statistically significant p-value. What they failed to disclose in their press release is that they ran analyses on 20 colours of jelly beans and obtained a “significant” p-value once.
If one has a large enough database, and runs enough analyses, one is almost certain to stumble on a relationship that is statistically significant. It is just a matter of numbers. Rolling the 20-sided die over and over is bound to produce “statistically significant’ results by chance alone. There are legitimate ways to test multiple hypotheses, but they require more stringent p-values to declare statistical significance. Had the jelly bean authors disclosed the multitude of analyses they performed, their results would have earned a yawn, not a headline.
This is closely related to the practice known as HARKing (hypothesising after results are known). In HARKing, investigators look at the data, run multiple analyses until they find something interesting (and probably statistically significant), then pretend that the results they found were what they had been looking for in the first place. If the Jelly Bean Study authors constructed a rationale that green jelly beans were uniquely suspected to cause acne, and reported their results as a confirmation of this hypothesis they would be guilty of HARKing.
Other researcher degrees of freedom
Researchers make many decisions when they perform a study. What kind of patients? What age range? How many? How long they will be followed? What parameters will be measured? At what points in time? If some patients miss exams or doses of medicine, how that be handled during data analysis? What statistical tests will be used? And on, and on. Collectively these are called researcher degrees of freedom. Ideally, these parameters will be defined before the study is begun. There can be a temptation to play with these parameters post hoc to create statistically significant outcomes.
An amusing but cautionary paper demonstrated that motivated manipulation of researcher degrees of freedom can dramatically alter research conclusions to such a degree that even absurd conclusions can be “proven” with statistical significance. In their example, they p-hacked data to prove that listening to certain music decreased the subjects age. These investigators also deserve credit for coining the term “p-hacking.”
P-hacking in action
Let’s say I run a startup company and have a promising vaccine to protect those bitten by the living dead from being transformed to zombies. I design a clinical trial comparing Zombivax vs placebo. The results of this study will determine the success or demise of my company. In the case of our study, the null hypothesis is that Zombivax and placebo are equally effective (or ineffective) in preventing zombie transformation. If our study shows Zombivax results superior to placebo with a P≤.05 we can then reject the null hypothesis and declare Zombivax effective. At the end of the study 43% of the Zombivax group became zombies compared to 68% of the Placebo group. How confident can I be that the difference between the treatments is real?
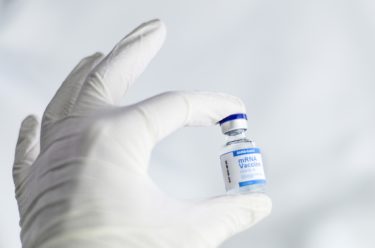
We analyse our results and achieve a p-value of 0.09. This means that IF Zombivax and placebo are equal (the null hypothesis) and we were able to run our clinical trial over and over again, we could expect, by chance alone, our results (43% for Zombivaz vs 68% for placebo) or a more extreme result 9% of the time. This result does not meet the prespecified threshold of P≤.05, so we would not be able to declare the effectiveness of Zombivax.
As CEO of the company, I am very disappointed that the clinical trial did not achieve statistical significance. I instruct my statisticians to go back and review the study design and analysis to see if any details were done improperly. They notice that some of the subjects in the Zomivax group missed one of 3 doses of the vaccine. If they omit those subjects from the analysis, the Zombivax group does a little better, now achieving a p-value of .07.
Next, they detect that the vaccine doesn’t seem to work as well in older subjects. If they limit the analysis to subjects 65 or younger, the results look much better, yielding a p-value of .04! As CEO I give my statisticians a bonus and issue a press-release declaring Zombivax a medical breakthrough.
What is wrong with exploring changes in the data and analyses to optimise the results? Once the data are known, there are many ways things can be adjusted and manipulated that will change the p-value. If one is so motivated it is possible to explore alternatives, accept those that move the results in a desirable direction and reject those that do not. This enables investigators to transform negative or borderline results into positive ones. This is the essence of p-hacking.
Using the 20-sided die as a metaphor, the clinical trial of Zombivax rolled the die. Unfortunately for our company, the die did not land on the ≤.05 side. It landed on the adjacent side for p-values between .05 and .10. What I instructed my statisticians to do is to kick, nudge, and tilt the table until the die rolls over to the desired result. If the die rolls in the wrong direction, they can just reset the die to the original roll and try something else. With enough motivation and creativity, it is likely that they can get the die to fall on the desired side and declare statistical significance.
If Zombivax was truly worthless, our clinical trial and subsequent p-hacking would be an example of a pure false positive result. If Zombivax was slightly effective, our p-hacking would be an example of “truth inflation,” transforming a small, statistically insignificant result into a larger, statistically significant one.
Conclusion: The significance of promoting the insignificant
The extent to which p-hacking can manufacture false positive results or exaggerate otherwise insignificant results is limited only by the p-hacker’s imagination and persistence. The results of p-hacking are much more consequential than simply padding an investigator’s resume or accelerating an academic promotion. Research resources are limited. There is not enough funding, laboratory space, investigator time, or patients to participate in clinical trials to investigate every hypothesis. P-hacked data leads to the misappropriation of resources to follow leads that appear promising, but ultimately cannot be replicated by investigators doing responsible research and appropriate analysis.
Provocative, p-hacked data can be the “shiny thing” that gets undeserved attention from the marketing teams, the press, and Wall Street. In medicine, compelling but dubiously-obtained results may be prematurely accepted into clinical practice. And within the CAM world, charlatans may can use sloppy research to promote worthless and irrational treatments.
There is no clear solution to solve the problem of p-hacking. Better education of investigators could reduce some of the more innocent instances. Greater transparency in reporting research results would disclose potential p-hacking. Deviations from planned data gathering and analysis plans should be disclosed and justified. For clinical trials, registries such as clinicaltrials.gov are intended to provide transparency in the conduct and reporting of clinical trials. Investigators are expected to “register” their studies in advance, including critical features of study design and data analysis. If used as intended, deviations from the registered and reported study details would be evident, and a red-flag for potential p-hacking.
Reducing or eliminating the reliance on p-values and the arbitrary dichotomy of statistically significant or insignificant results has been proposed by the American Statistical Association.
Greater understanding of p-hacking among investigators, journals, peer-reviewers, and consumers of scientific literature will promote more responsible research methodologies and analyses.
Further reading:
- This article is derived from one previously published at Science Based Medicine.
- Jonathan Jarry wrote a very nice shaggy dog story illustrating p-hacking.
- A more technical and more comprehensive discussion of p-hacking can be found here, highly recommended reading. Big little lies: a compendium and simulation of p-hacking strategies.